
Second, the quality of lung sound is easily affected by the patient’s effort to breathe. First, the lungs are much larger than the heart and lung sounds should be recorded at multiple sites of both lungs for an accurate analysis. Auscultation of the lung is different from that of the heart in some aspects. AI was used to distinguish different murmurs and diagnose valvular and congenital heart diseases 21. There are several published studies on AI-assisted auscultation of heart and lung sounds 13, 17, 18, 19, 20. Recently, deep learning is widely applied to some medical fields including a chest x-ray or electroencephalogram analysis 15, 16. It can be used to screen and monitor patients with various pulmonary diseases including asthma, COPD and pneumonia 13, 14. AI-assisted auscultation can help a proper diagnosis of respiratory disease and identify patients in need of emergency treatment.
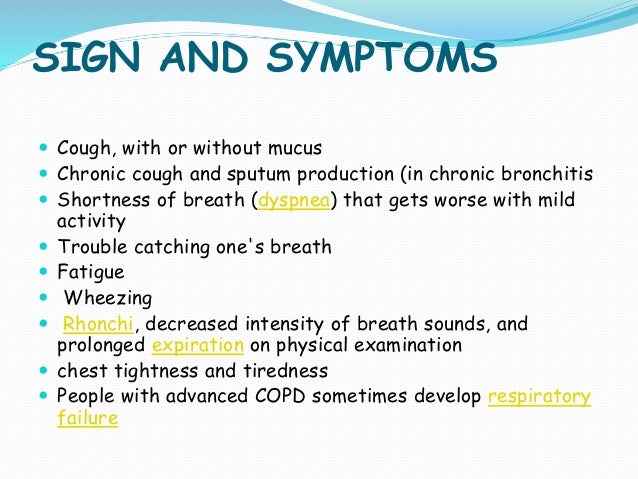

These limitations of auscultation raised the need to develop a standardized system that can classify accurately respiratory sounds using artificial intelligence (AI). reported significant inter-observer differences in terms of discriminating expiratory rhonchi and low-pitched wheezes from other sounds, potentially compromising diagnosis and treatment 12.

found that hospital trainees misidentified about half of all pulmonary sounds, as did medical students 11. Rhonchi are musical low-pitched sounds similar to snores, usually indicating secretions in the airway, and are often cleared by coughing 1.Īlthough auscultation has many advantages, the ability to analyze respiratory sounds among clinicians varies greatly depending on individual clinical experiences 6, 10. Wheezes are musical high-pitched sounds associated with airway diseases such as asthma and chronic obstructive pulmonary disease (COPD). Crackles, which are short, explosive, and non-musical, are produced by patients with parenchymal lung diseases such as pneumonia, interstitial pulmonary fibrosis (IPF), and pulmonary edema 1, 8, 9. Crackles, wheezes and rhonchi are the most commonly found among them, and detecting those sounds greatly aids the diagnosis of pulmonary diseases 6, 7. Abnormal lung sounds include crackles, wheezes, rhonchi, stridor, and pleural friction rubs (Table 1). Recent electronic stethoscopes have rendered lung sounds recordable, and it facilitated the studies of automatically analyzing lung sounds 4, 5. Auscultation is non-invasive, real-time, inexpensive, and very informative 1, 2, 3. The stethoscope has been considered as an invaluable diagnostic tool ever since it was invented in the early 1800s. Our deep learning-based classification would be able to complement the inaccuracies of clinicians' auscultation, and it may aid in the rapid diagnosis and appropriate treatment of respiratory diseases. On the other hand, as a result of respiratory sound classification by different groups showed varying degree in terms of accuracy the overall accuracies were 60.3% for medical students, 53.4% for interns, 68.8% for residents, and 80.1% for fellows. It further classified abnormal lung sounds into crackles, wheezes, or rhonchi with an overall accuracy of 85.7% and a mean AUC of 0.92. It detected abnormal sounds with an accuracy of 86.5% and the area under the ROC curve (AUC) of 0.93. We developed the predictive model for respiratory sound classification combining pretrained image feature extractor of series, respiratory sound, and CNN classifier. We utilized deep learning convolutional neural network (CNN) to categorize 1918 respiratory sounds (normal, crackles, wheezes, rhonchi) recorded in the clinical setting. To overcome such limitations, we tried to develop an automated classification of breath sounds.
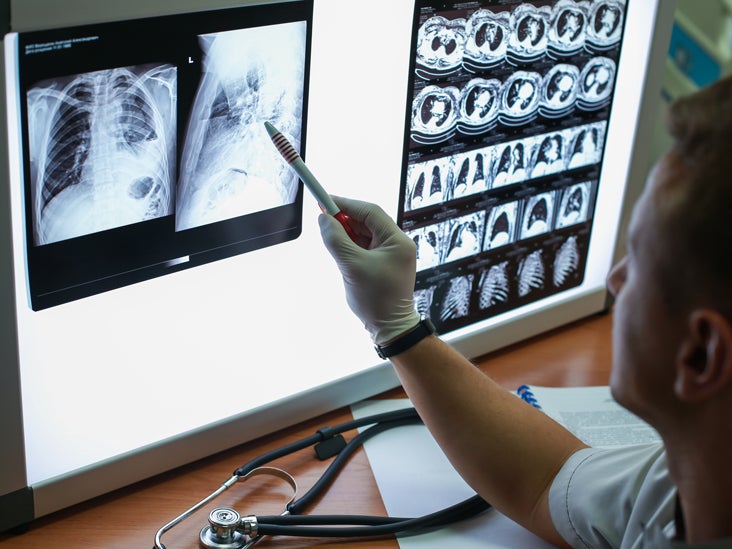
However, accurate interpretation of respiratory sounds requires clinician’s considerable expertise, so trainees such as interns and residents sometimes misidentify respiratory sounds. Detection of abnormal respiratory sounds with a stethoscope is important in diagnosing respiratory diseases and providing first aid. Auscultation has been essential part of the physical examination this is non-invasive, real-time, and very informative.
